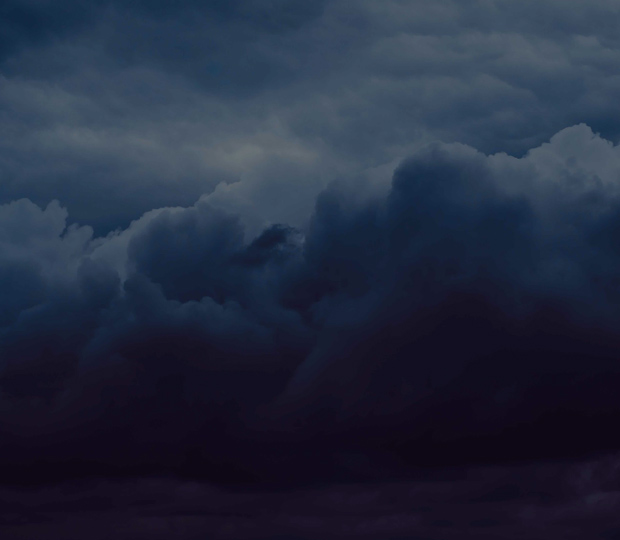
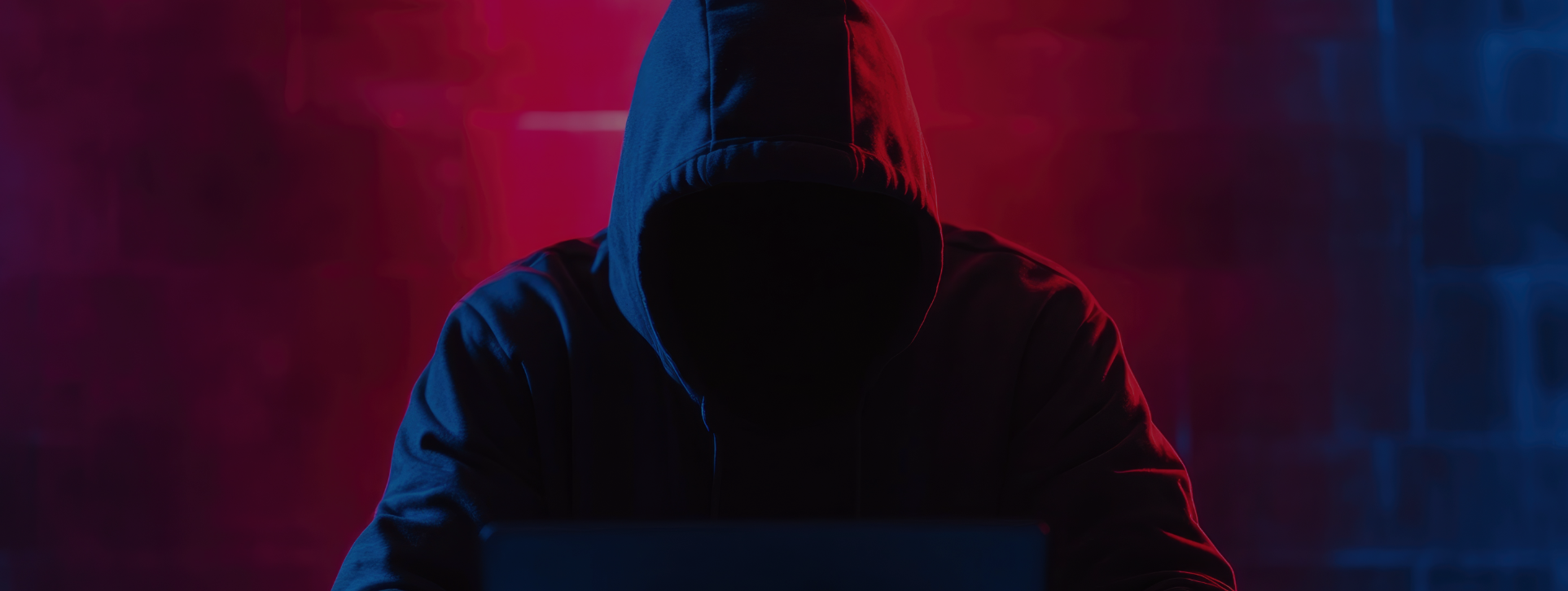
Cybersecurity: New Breed of AI Threat
How emerging AI-powered attacks are redefining the threat landscape
Share this
Publish date: 07.05.25
The cybersecurity domain is experiencing a seismic shift — not just in scale, but in the very nature of cyber threats. As organisations race to deploy AI tools for defence, threat actors are doing the same on the offensive front. What we now face is a new breed of cyberattacks: AI-powered, highly adaptive, and rapidly scalable.
This isn’t a just a purely theoretical future state of threats we’re looking at. AI will account for 75 percent of cyberattacks by the close of 2025, a new Gartner estimate implies – the deployment of AI in cybercrime is already completely upending the threat landscape. From autonomous phishing to deepfake-driven impersonation and machine-assisted malware evolution, attackers are using artificial intelligence to outpace traditional security tools and operational policies. The challenge ahead is not only technical, but architectural and strategic.
Let’s explore how AI is fuelling the next generation of cyber threats — and what IT leaders must do to respond.
Phishing Has Weaponised Generative AI
Phishing has historically been plagued by tell-tale signs, making it easy for users to spot: bad grammar, inconsistent formatting, and a lack of any real personalisation. But with the advent of generative AI tools, this is no longer the case. Attackers now use large language models to craft grammatically accurate, contextually relevant, and highly personalised phishing emails. These messages often mimic an organisation’s internal tone of voice and vocabulary, using scraped data from social media or company websites to sound like they come from trusted colleagues or senior leadership.
This isn’t limited to email either — adversaries are now extending phishing attacks into collaboration platforms like Microsoft Teams and Slack, meaning that social engineering attacks are appearing across a plethora of new platforms, catching users unaware.
Traditional anti-phishing filters, which rely on keyword heuristics or known malicious domains, are no longer sufficient. Your security tools need to include behavioural and context-aware analysis, using natural language processing (NLP) and historical communication analysis to detect anomalies in sender behaviour, message tone, and interaction patterns.
Deepfakes and Synthetic Identity
Another perhaps extreme example is the operational use of deepfake technology. AI models can now synthesise convincing voices and faces — and this technology is being used in active fraud campaigns. Attackers have been documented using voice-cloned phone calls from board members or business owners to manipulate employees into transferring money or disclosing login credentials. In some cases, video deepfakes have been created to deliver false directives in executive impersonation scams.
These attacks are particularly dangerous because they exploit hardwired trust in identity and authority, and they’re extremely difficult to detect in real time. Traditional identity verification systems — like voice recognition or facial biometrics — are increasingly vulnerable.
Organisations must now consider multi-factor authentication for sensitive verbal or video communications, including challenge-response protocols that validate identity beyond voice or appearance. Training staff to identify cues of synthetic media, such as uncanny audio latency or subtle visual artifacts, is becoming essential.
AI-Augmented Malware Is Becoming Polymorphic and Context-Aware
On the malware front, attackers are leveraging AI to create polymorphic payloads that mutate in response to your defensive measures. Using reinforcement learning, malware can now test and adjust its behaviour in real time based on feedback from the target environment. For example, some payloads delay execution or alter code paths when they detect virtual environments or sandboxes — a tactic designed to evade detection by automated analysis systems.
Worse still, AI models are now being used to optimise malware distribution based on endpoint telemetry, targeting vulnerable machines with precision and changing delivery tactics as conditions shift.
To counter these threats, endpoint detection and response (EDR) platforms must evolve to include kernel-level behavioural monitoring and process tree analysis — not just static scanning. Security teams need solutions that can spot anomalies in process behaviour, file access patterns, and memory usage, regardless of how the malware has been obfuscated.
Automated Reconnaissance is Speeding up Attacks
Attackers are also using AI to drastically accelerate reconnaissance and targeting. In the past, profiling an organisation required manual research. Now, attackers can deploy bots that scrape LinkedIn, GitHub, company websites, and breach databases to build detailed profiles of employees and infrastructure. These bots can correlate job titles, projects, tech stacks, and exposed services to determine the best path of attack.
This automation reduces the attack lifecycle from days or weeks to hours. More importantly, it enables hyper-personalised attacks at scale, something that was previously cost or resource prohibitive.
To defend against this, organisations must minimise their external attack surface. This includes obscuring unnecessary metadata, enforcing minimal information disclosure on public-facing systems, and implementing external threat surface management tools that identify and monitor exposed assets in real time.
LLM-Assisted Exploit Discovery Is Democratising Vulnerability Research
One of the most disruptive uses of AI is in automated exploit discovery. Generative AI models trained on open-source code repositories and vulnerability databases can now assist attackers in locating misconfigurations, insecure logic flows, and outdated libraries in applications. Combined with fuzzing engines and static analysis tools, attackers can rapidly identify and chain together sets of vulnerabilities — even in moderately secure IT environments.
This brings zero-day research capabilities within reach of lower-ability threat actors, significantly raising the bar for defenders.
To mitigate this, organisations must shift security left in the development lifecycle. Tools that integrate into CI/CD pipelines and apply AI-based static and dynamic code analysis can flag risky constructs before deployment. Moreover, software composition analysis (SCA) should be used to track third-party component risk and license compliance.
Prompt Injection and AI Hijacking Are Emerging Attack Classes
As more organisations integrate AI into their workflows — including customer service bots, internal assistants, and developer copilots — they inadvertently introduce a new threat: prompt injection. Attackers are actively experimenting with methods to subvert AI responses, manipulate decision logic, or exfiltrate sensitive data.
For instance, malicious inputs can be designed to trick an AI model into disclosing confidential context, bypassing filters, or executing unintended actions. Worse, these attacks are hard to detect because the payload is embedded in natural language, not code.
Defending against this requires a layered AI security strategy: input sanitisation, output filtering, context isolation, and auditing of AI behaviour. AI systems should be treated like any other production system — with access controls, logging, and vulnerability assessments.
AI is Now an Adversary
We’re no longer fighting static, traditional, rule-based threats. The adversary is now intelligent, adaptive, and autonomous. The defensive posture that once relied on signature-based detection, periodic audits, and siloed tools is rapidly becoming obsolete.
What’s needed is a modernised cybersecurity architecture that includes:
- AI-driven threat detection that leverages behavioural analysis and anomaly detection.
- Automation of incident response to reduce dwell time and mitigate lateral movement.
- Zero Trust principles, including continuous verification of users, devices, and access.
- Cross-domain telemetry correlation, bringing together signals from endpoints, identity, network, and cloud.
As AI-driven threats evolve in complexity and speed, organizations need more than reactive tools — they need a proactive, unified defence strategy. Xeretec’s Cyber Security and Advanced Threat Protection portfolio is designed specifically for this new landscape. We combine cutting-edge threat intelligence, AI-driven anomaly detection, and real-time incident response to secure your endpoints, users, and cloud environments. Whether you’re looking to strengthen your phishing defences, implement Zero Trust access controls, or monitor for AI-powered malware attacks, Xeretec delivers enterprise-grade protection with seamless integration into your existing architecture.
Contact us today to assess your current exposure and learn how Xeretec can help you stay one step ahead of AI-driven threats.
So, What Exactly Is An AI PC?
Read more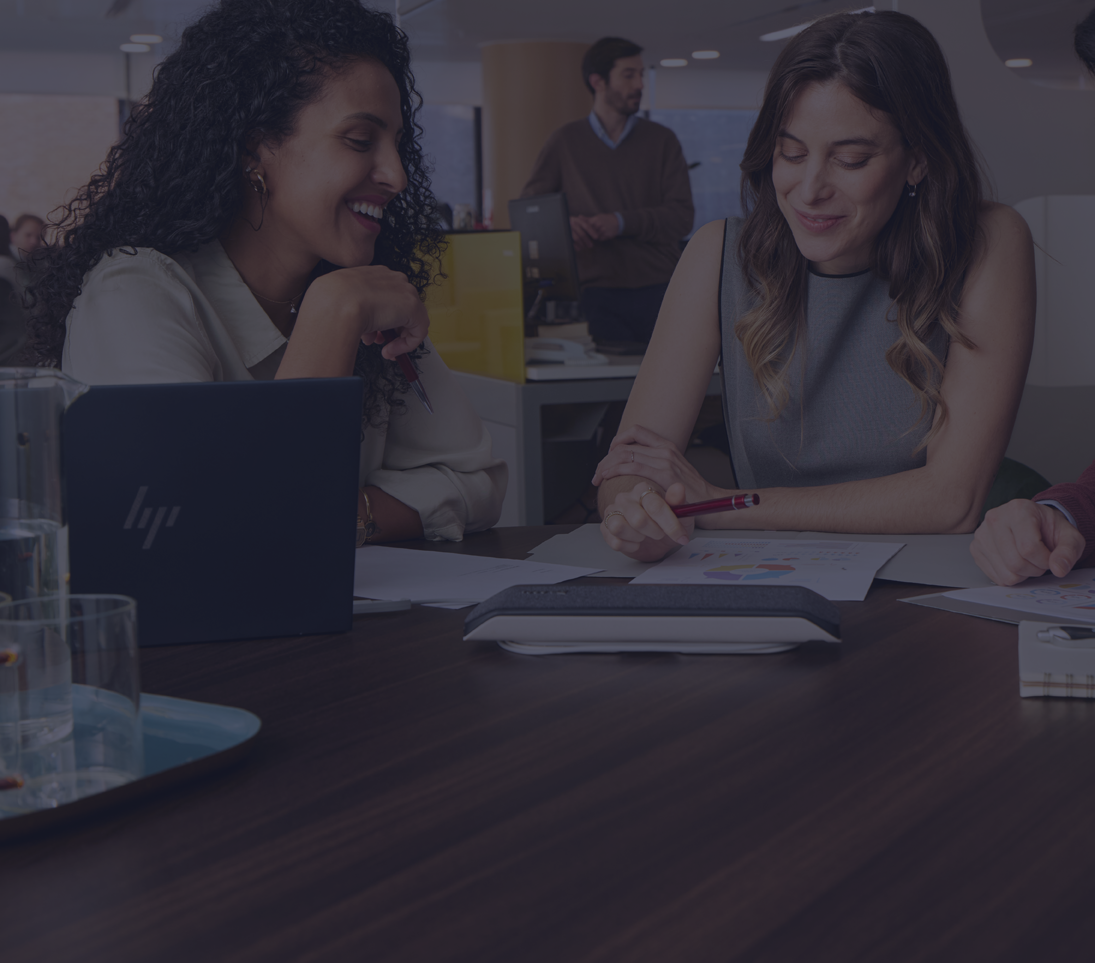